TERESAÂ CHANG
Geospatial Data Enthusiast | Transportation Planning | Urban Technologies | Sustainability Â
City Planning @ Penn | Civic Data Analytics Intern @ Alta Planning + Design
As a Master of City Planning student at the University of Pennsylvania, my passion lies in the intersection of spatial data analytics, transportation, and climate change. I have focused on Smart Cities topics and established my spatial data analysis ability, utilizing sensors and data to improve urban infrastructure and decision-making while ensuring civic engagement and equity. I'm also passionate about public transit, active transportation, and all kinds of new shared mobility.
My background in environmental engineering has given me a strong foundation in sustainability and water management, and I am interested in leveraging environmental technology to mitigate climate change and promote a greener future.
PROJECTS
Take a look at the exciting projects I have been working on!
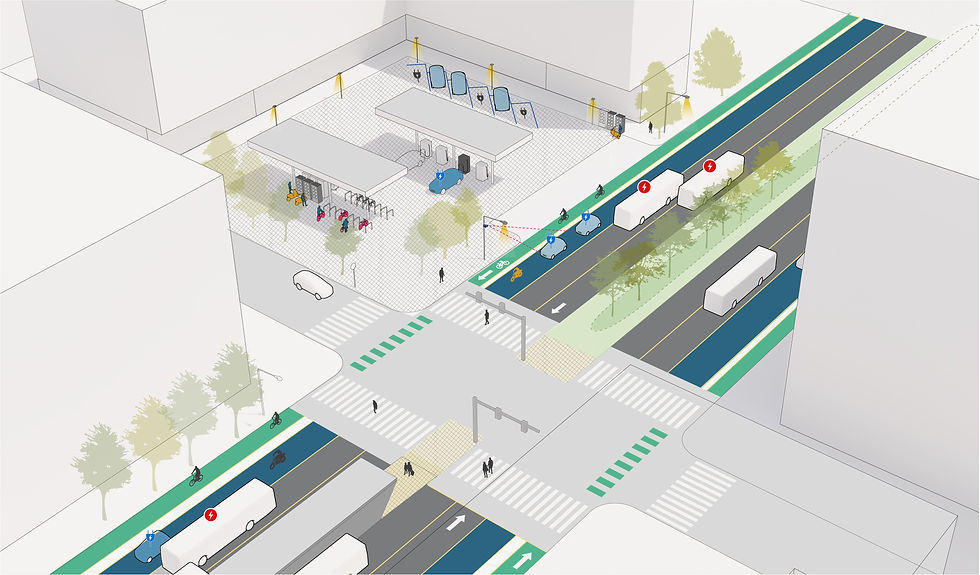
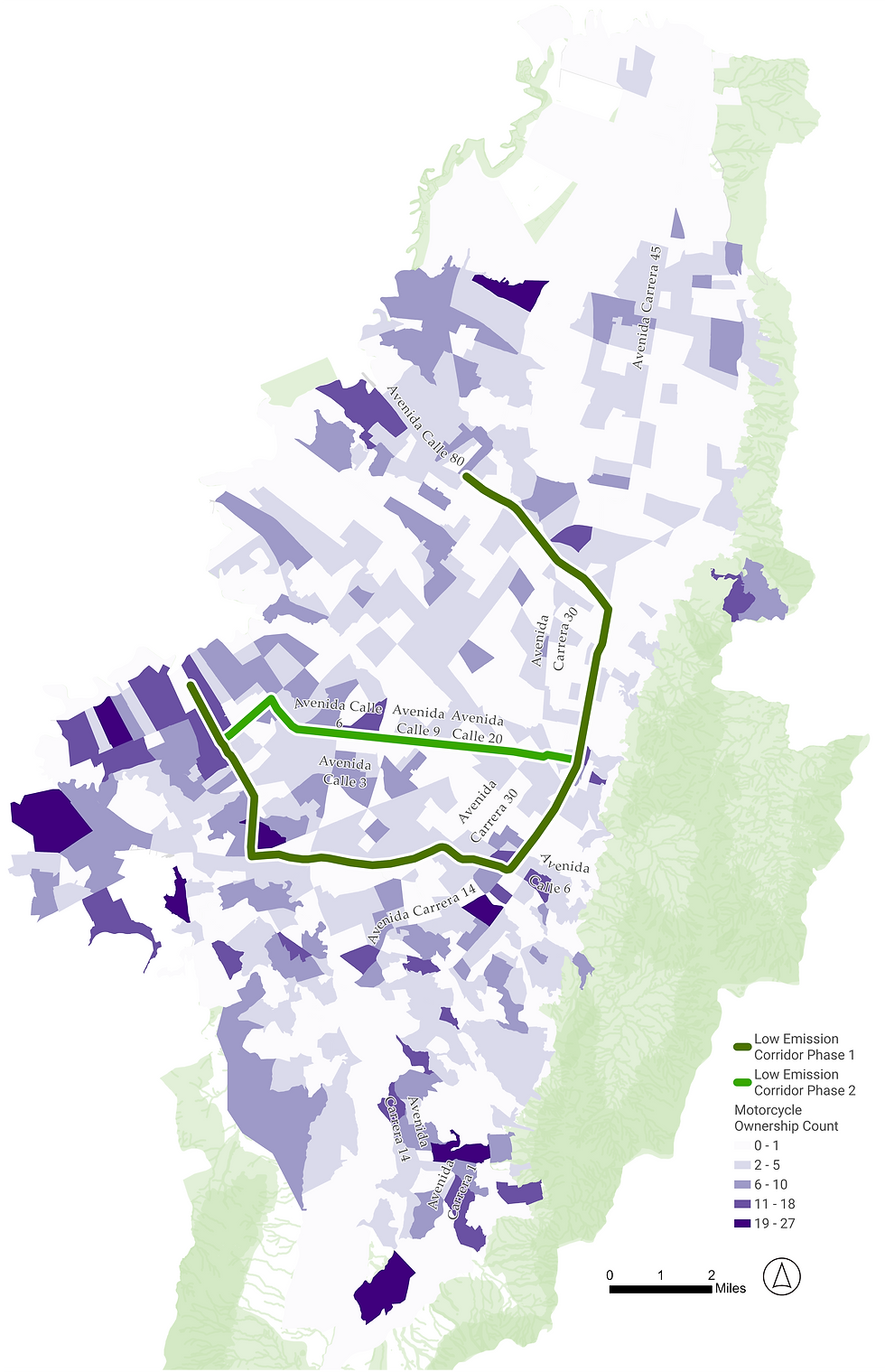
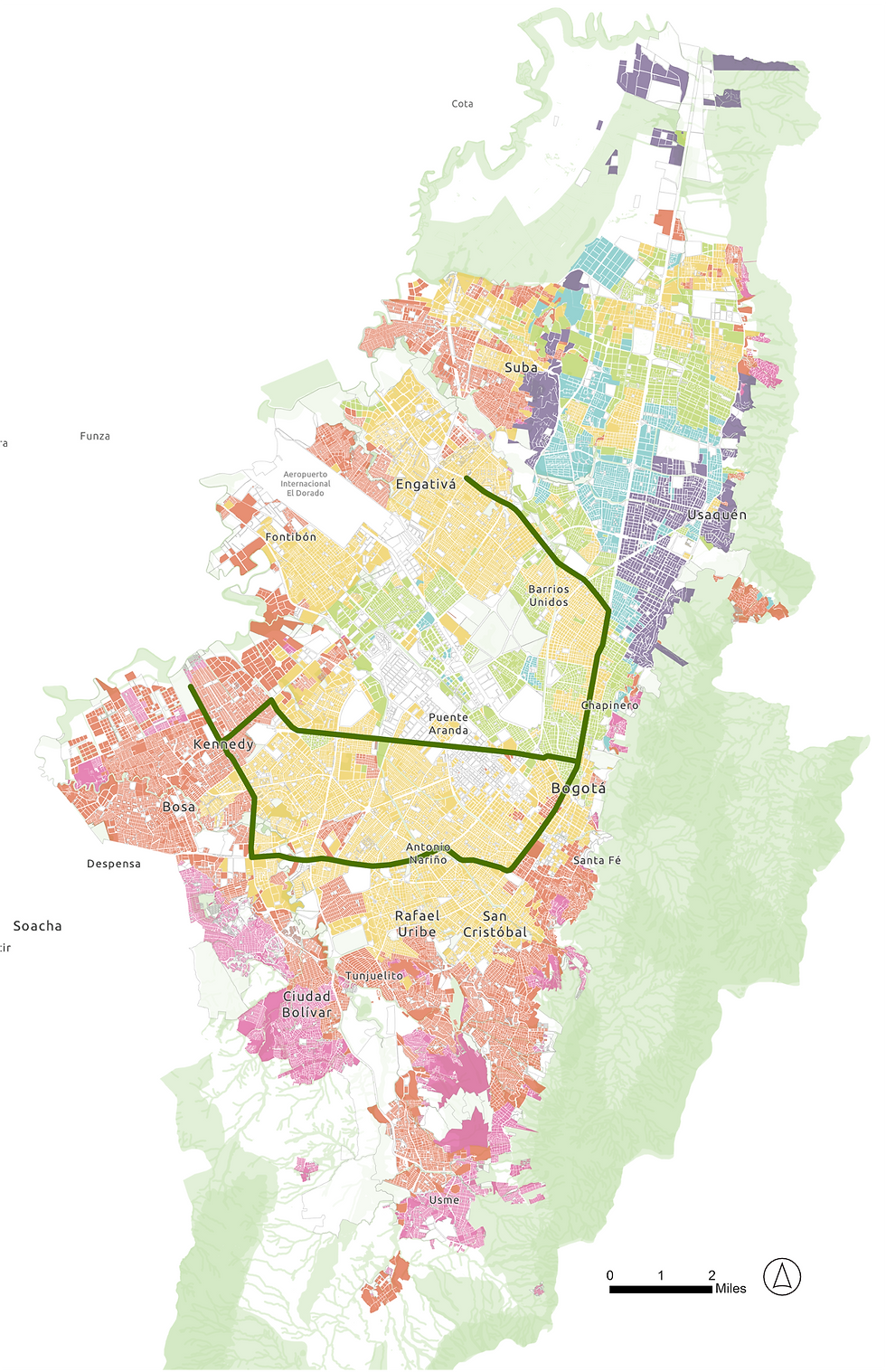
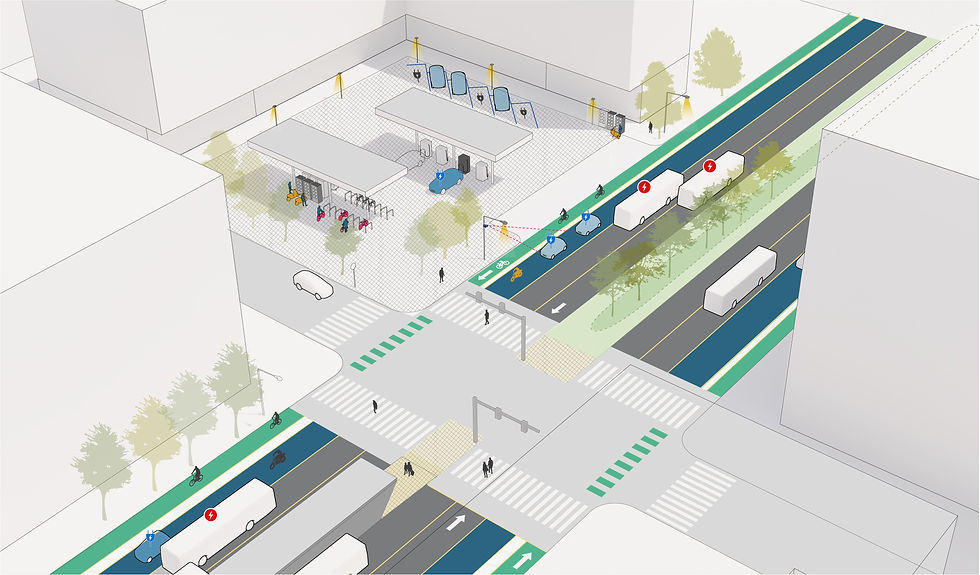

The bikeshed from the original station entrance.

Adding another entrance across the CSX rail.
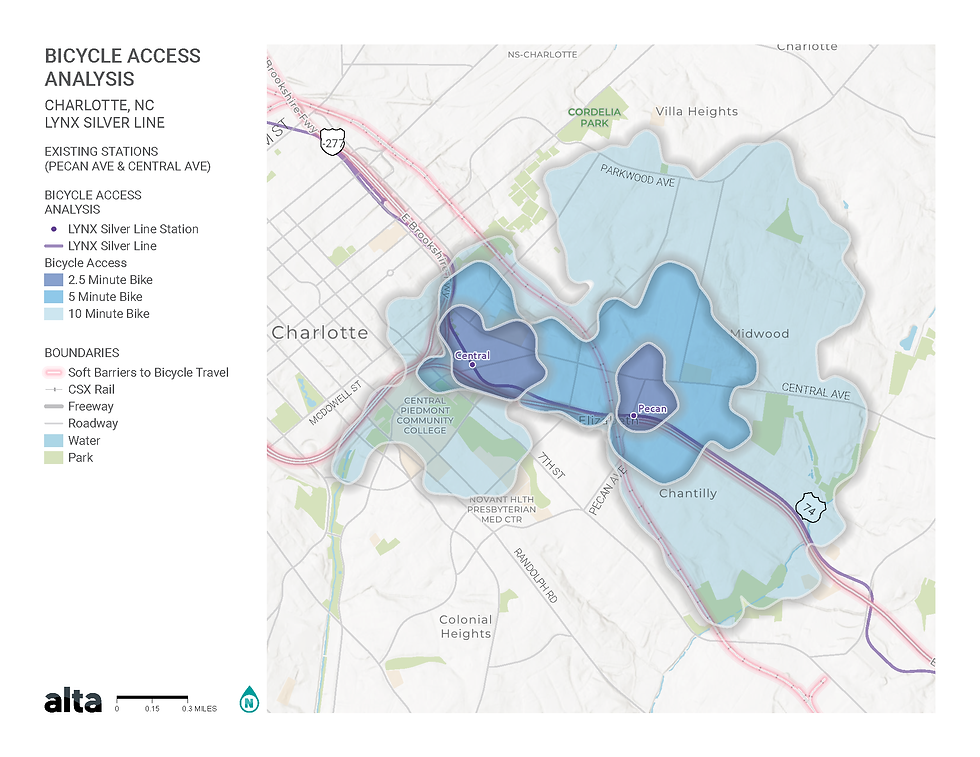
Adding another entrance across CSX rail.

The bikeshed from the original station entrance.



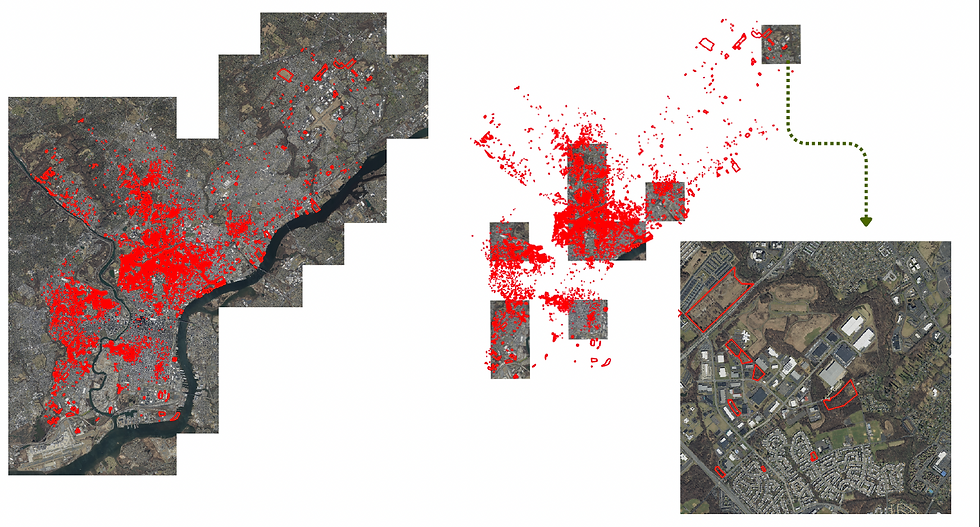
Ten tiles imagery and vacant parcels shape file prepared for labeling.
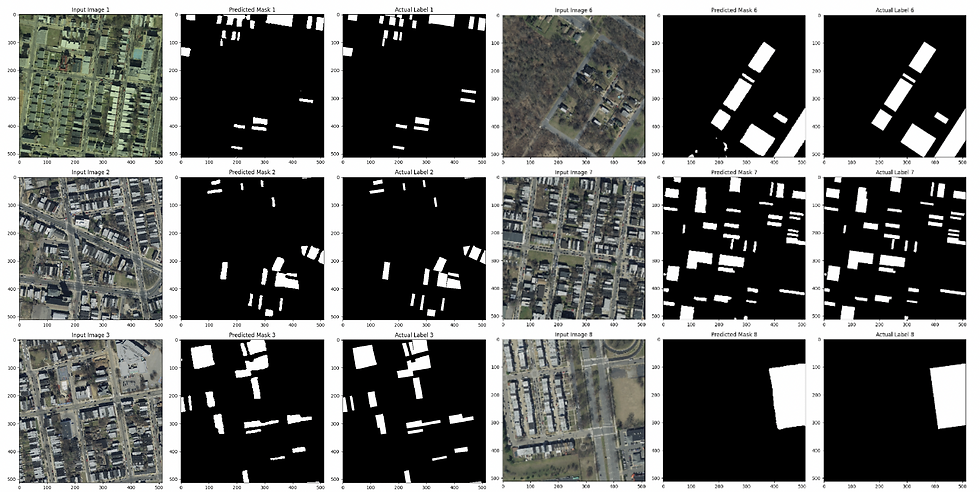
Prediction Masks
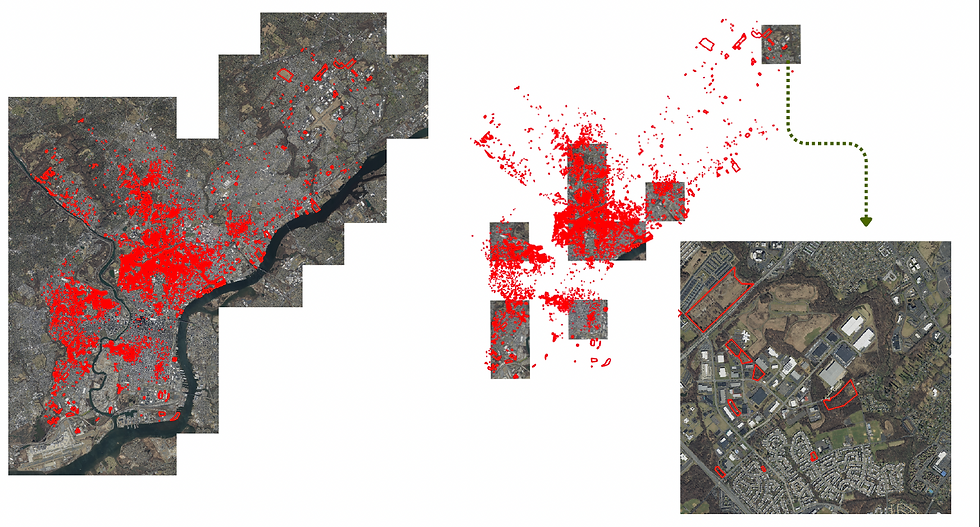
Ten tiles imagery and vacant parcels shape file prepared for labeling.

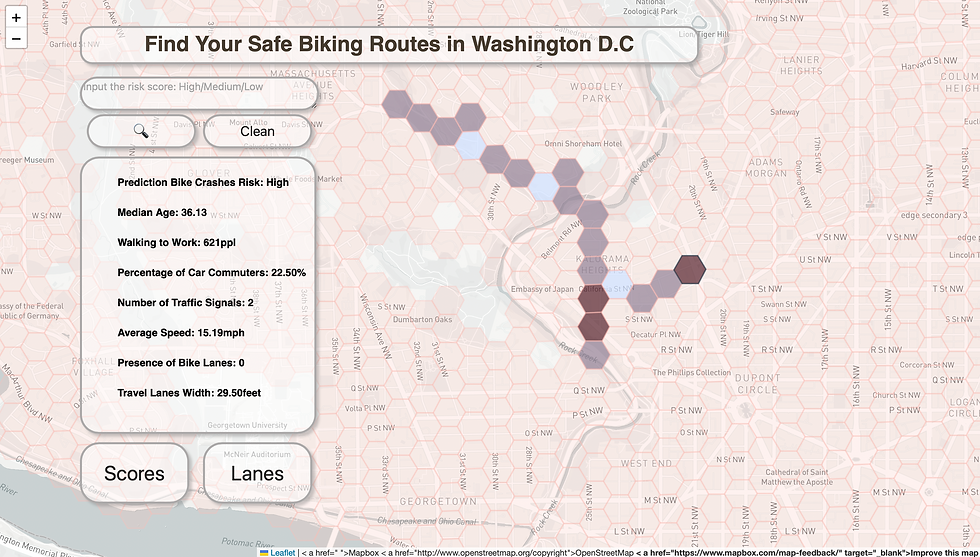

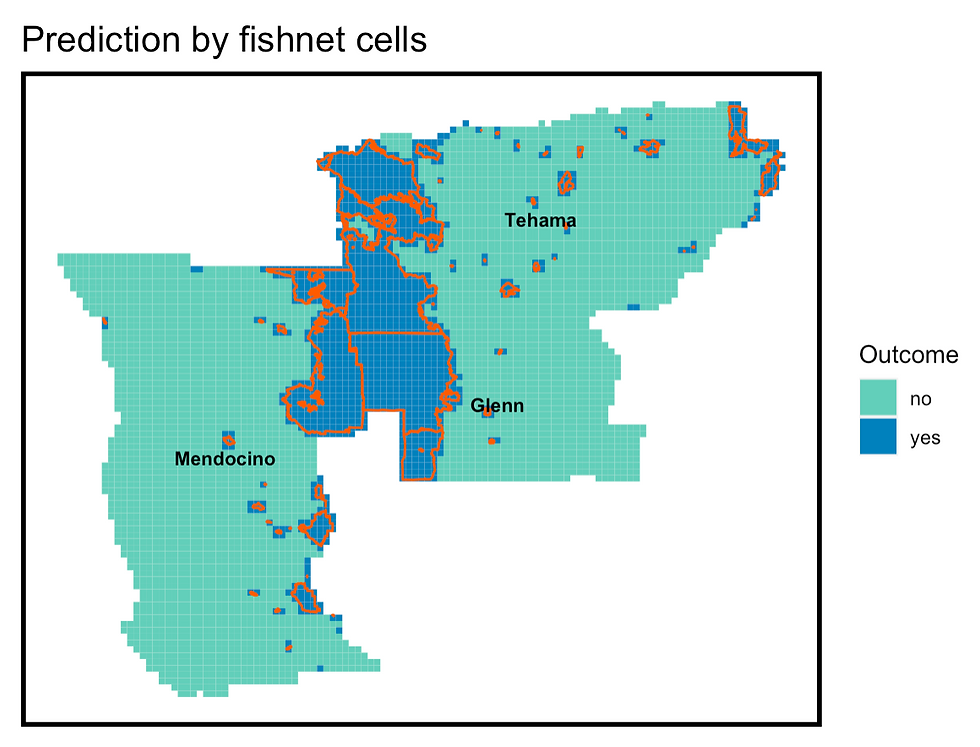


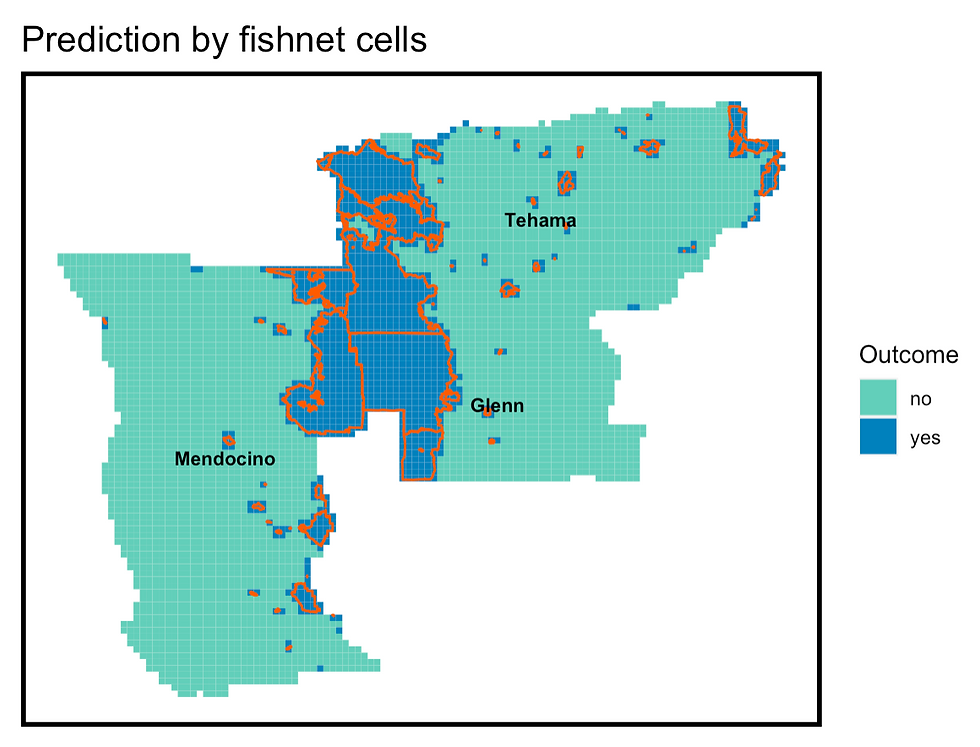
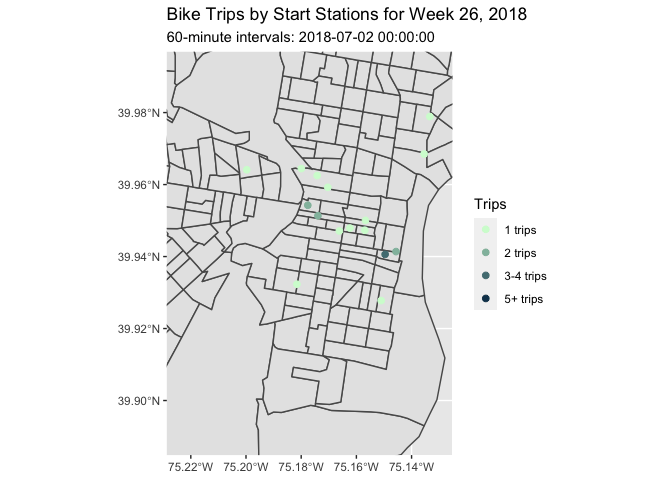

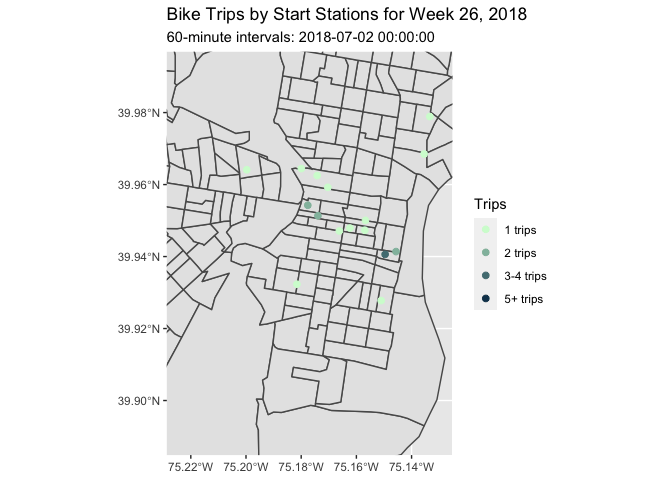
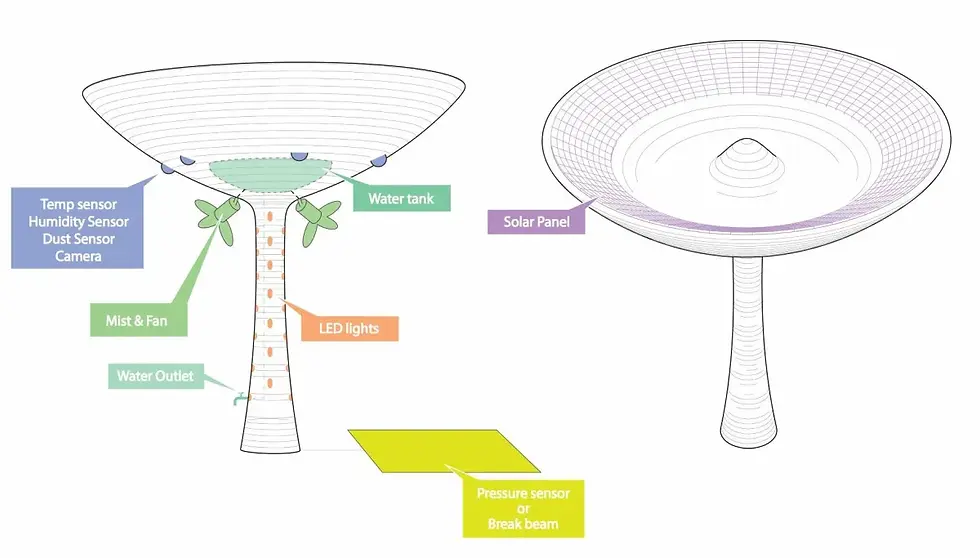
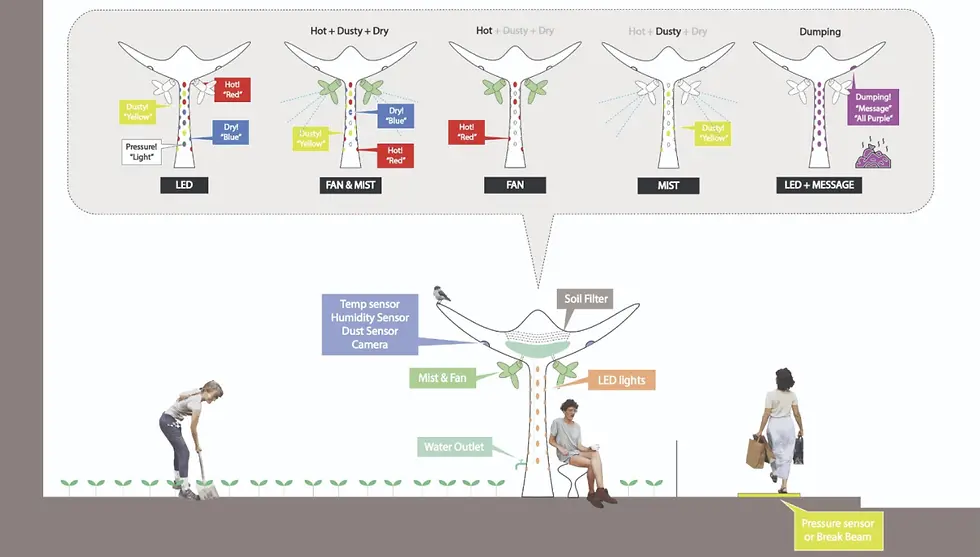
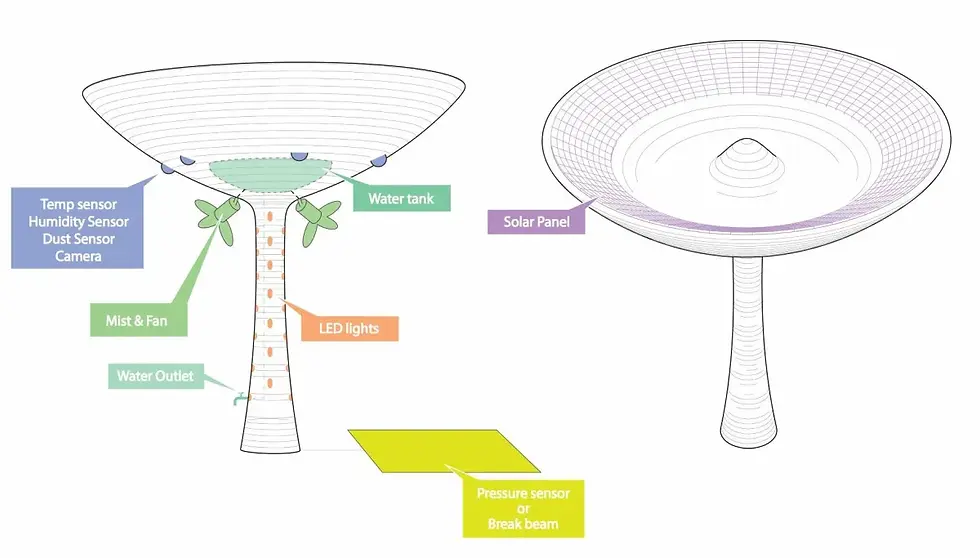
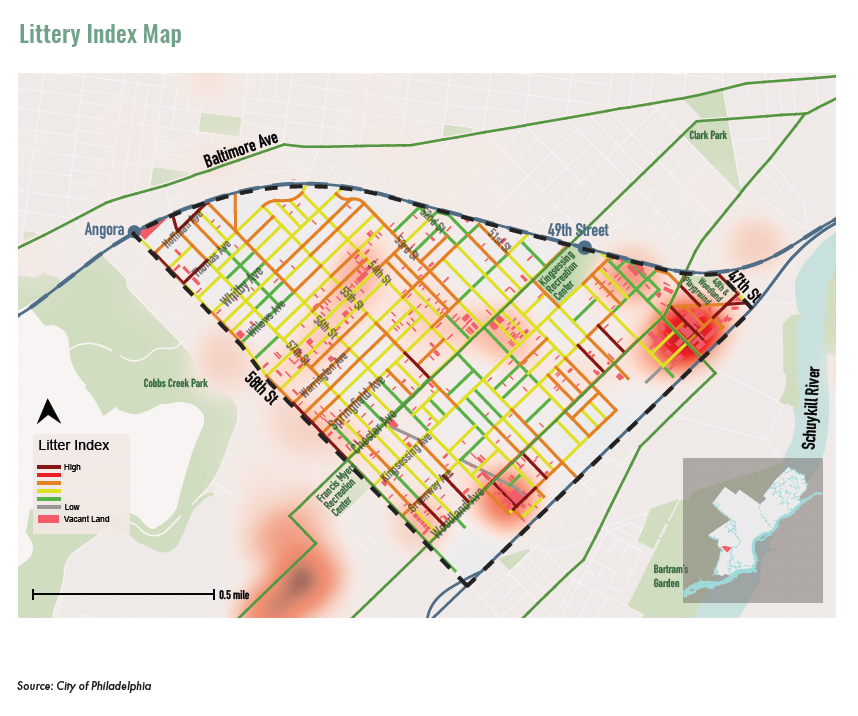
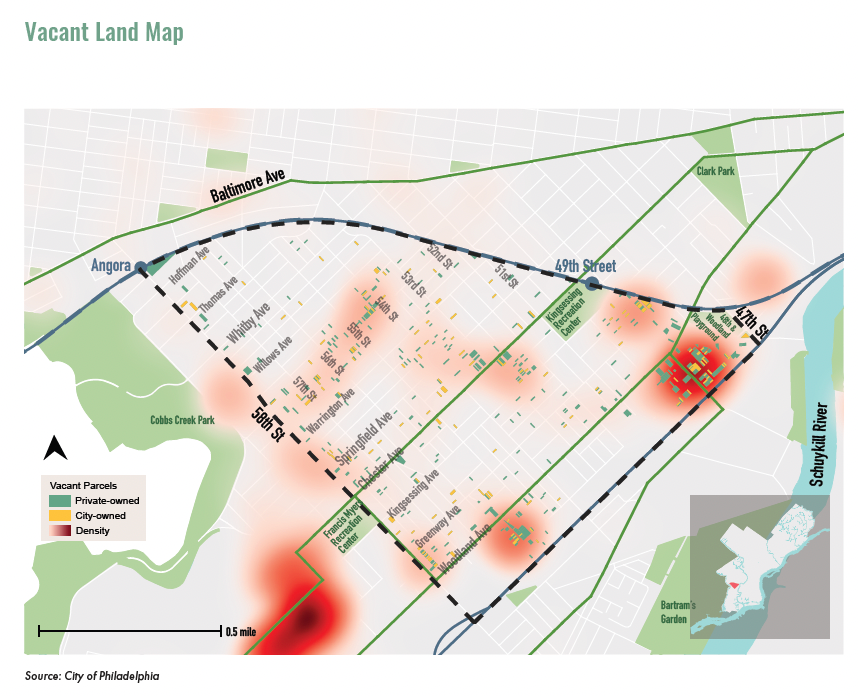

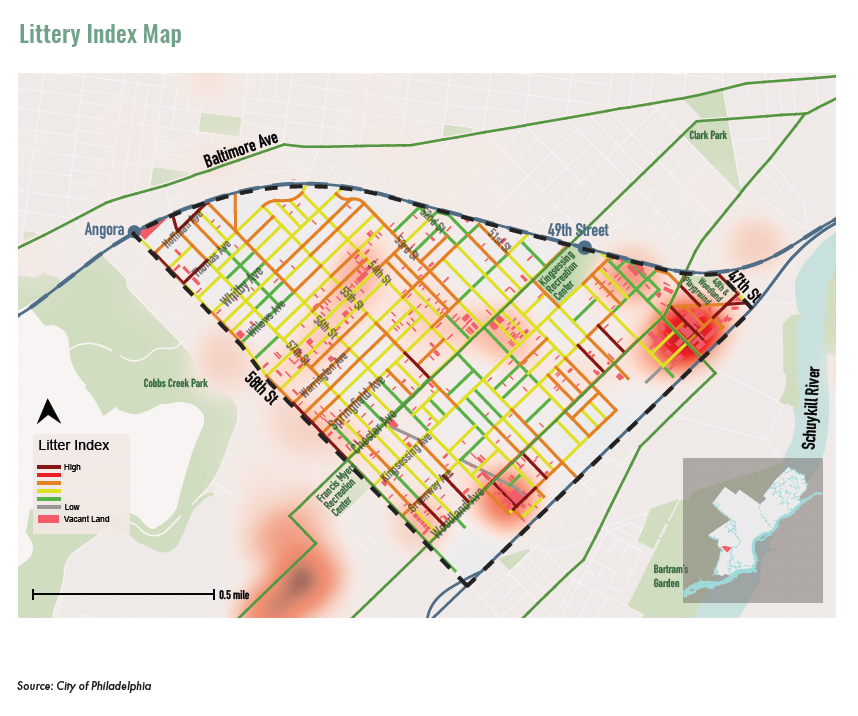
KINGSESSING NEIGHBORHOOD PLAN
Planning | GIS | Design
In the Kingsessing Neighborhood Plan, I am in charge of the mapping style and spatial analysis of topics including vacant land analysis, crime incidents analysis, litter index analysis, and housing development analysis, among others. Click "Learn More" to see my work and design!
​
HEALTHY NOOK: MONITORING AND REACTING TO ENVIRONMENTAL CONDITIONS IN COMMUNITY GARDENS
Arduino | Sensors | C++ | Smart Cities
Healthy Nook is an installation that aims to improve health and environmental conditions for community gardeners in the field. It features:
Cameras and LED lights to discourage illegal dumping and optimize dumping management.
Stormwater collection tank to increase water accessibility
Mister and fans to cool and weigh down harmful air particles in the gardening area
Overall, it will make community gardening experience more enjoyable, comfortable, and less physically demanding!
Click to see our prototype!
SPACE-TIME PREDICTION OF BIKE SHARE DEMAND IN PHILADELPHIA
R | Machine Learning | Data Analysis
Bike share has become more and more popular in big cities since it is convenient for us to rent bike for short commuting from point to point. However, it is crucial for share bikes stations to balance their bike demand. It is important to come up with a re-balancing strategy to better allocate the share bike distribution and the prediction of a share bike demand either spatially or temporally. In this report, I built a prediction model for bike sharing demand based on Philadelphia’s Indego bike share system. The model has a moderate accuracy and generalizability to predict the demand temporally and spatially, which can further utilize in the re-balancing plans for Indego to predict each stations demand two weeks earlier and make plans for redistributing the bikes from station to station
FORECASTING WILDFIRE RISK IN NORTHERN CALIFORNIA
R | Machine Learning |Â Data Analysis
With climate change, wildfire has become more destructive to personal and property safety. There were more than one million fires that resulted in thousands of deaths and injuries in the U.S. And it is only for the year 2021. Among them, California is the most risky state. This project aim to predict the wildfire spatial distribution to let people avoid the potential area using machine learning model. We selected Tehama, Glenn, and Mendocino County in California as our testbed. We hope to partner with Google Maps to add a layer of wildfire prediction to the app to alert and optimize route planning.Â
As a result, Sensitivity, which is the ability to predict true positive outcomes is 58%, and specificity, which means the ability to predict true negative outcome, is 96%.
FIND YOUR SAFE BIKING ROUTES IN WASHINGTON, D.C.
Cloud Computing | Google Cloud |Â Python | JavaScript
This project establishes a website that provides bikers with a service to identify safer biking areas in Washington, D.C., and enables policymakers to identify high-risk biking areas and provide effective solutions. Users can interact with our map on the website to explore the biking risk scores and other basic information about their potential biking areas and design their own biking routes accordingly.
IDENTIFYING VACANT LOTS INÂ PHILADELPHIAÂ USING SATELLITE IMAGERY ANDÂ DEEP LEARNING
Python | Remote Sensing | Deep Learning
This project aims to use deep learning models to automate the identification of vacant parcels using satellite imagery, providing valuable insights into the distribution of unused land and supporting further urban planning processes. We employed three main models for image segmentation tasks, including U-Net, modified U-Net incorporating the pre-trained MobileNetV2 as its encoder, and a U-Net architecture with an EfficientNetB0 backbone. As a result, Model 2 demonstrates superior results across all considered metrics, including the highest training accuracy of 99.2%.
​
TRAVEL-RELATED PHYSICAL ACTIVITY HOURS ANALYSIS
Python | Data Visualization | @Alta
This R&D project at Alta involved evaluating the intersection of public health and mobility by leveraging Replica's Activity-based models to provide insights into the time spent walking and biking on utilitarian trips.Â
The Python script I created calculates the travel-related physical activity hours, which can provide evidence to promote health and recommend active transportation modes to increase the activity hours of each adult and achieve the CDC's recommended 150 minutes of physical activity per week. It is important to note that this analysis is based on modeled typical travel trips from Replica, not including looping trips. The output includes three charts: weekly physical activity hours by block group, weekly physical activity hours by traveler, and a bar chart of physical activity by race/ethnicity.
WALK AND BIKESHED ANALYSIS FOR A LIGHT RAIL STATION
ArcGISÂ Network Analyst | Python | OpenStreetMap | Cartography | @Alta
This project analyzes the potential increase in pedestrian and bicycle access resulting from the proposed light rail station entrance and a rail trail. The analysis involved extracting data from the OpenStreetMap network and calculating the Level of Traffic Stress (LTS) based on network characteristics. Additionally, I assessed population and employment coverage within each shed using ACS data. Taking the bikeshed as an example, the results reveal a population increase of up to 57% and employment surge of up to 537% within a 2.5-minute biking distance, attributed to the introduction of an additional entrance across the CSX rail, which previously hindered east-to-west access. Furthermore, the construction of the rail trail demonstrates a substantial increase in benefits, extending access to a larger population and employment opportunities.
LOW EMISSION CORRIDORS - STUDIO
Planning Studio | GIS | Graphic Design
This project is one of the proposals of the Studio project in Bogota, Colombia, addressing challenges in the world's largest high-capacity BRT system and exploring the potential economic impact of transit-oriented development. The low-emission corridor project introduces three new street designs with EV-only lanes and incorporates e-motorcycle systems, considering the high motorcycle ownership in Bogota. Additionally, I led efforts to examine EV purchase and usage incentives and introduced the concept of retrofitting mobility hubs along the corridor. Identifying the low-emission corridor involved a suitability model, considering factors such as air quality, proximity to amenities, motor vehicle ownership, and residential zones. This pioneering project has the potential to serve as a model for countries with similar characteristics, facilitating the transition to electrification and emissions reduction.
Feel free to contact me to work on thrilling projects in planning/geospatial data analysis together.